Introduction to Machine Learning
Explore Introduction to Machine Learning: Linear Regression, Hackathon, Kaggle, Supervised learning, Regression, Unsupervised Learning, Recommender System, & ML on Cloud. Unlock the potential of data-driven intelligence!
Instructor:
Dr. Abhinanda SarkarRatings
Level
Learning hours
Learners
Skills you’ll Learn
About this course
Machine Learning is a go-along domain with Artificial Intelligence in Computer Science and Technology, which deals with training the machines with previously trained models. The system self-learns the process improves without munch of human intervention required. With the world driven by advancements in Artificial Intelligence and its technologies today, Machine Learning is gradually making its stand in various fields. Machine Learning is also one of the most sought job choices, and thus many aspirants learn it. This course introduces you to the world to Machine Learning. You will undersand niche concepts such as Supervised and Unsupervised learning, Regression and Classification. This course will educate you about different platforms where you can participate in competitions conducted world wide such as hackathon, kaggle. You also get to know the concepts behind recommendation systems and how ML on cloud is emerging.
The faculty for the course is Dr. Abhinanda Sarkar, Ph.D. from Stanford University and Ex-Faculty MIT, is Academic Director at Great Learning for Data Science and Machine Learning Programs.
Check out our PG Course in Machine learning Today.
Course Outline
Data is the soul of Machine Learning, and there are specific methods to deal with it efficiently. This module first introduces Machine Learning and talks about the mathematical procedures involved. You will learn about supervised and unsupervised learning, Data Science Machine Learning steps, linear regression, Pearson's coefficient, best fit line, and coefficient of determinant. Lastly, you will be going through a case study to help you effectively comprehend Machine Learning concepts.
Machine learning algorithms involve seven steps: Collect data, Prepare the data, Choose the model, Train the machine model, Evaluation, Parameter tuning, Prediction or Inference.
Kaggle supports a no-setup, customizable Jupyter Notebooks environment. It helps access free GPUs and a vast community published code and data repository. Hackathons are designed sprint-like events that focus on creating a functioning software or hardware where programmers, graphic designers, interface designers, project managers, domain experts, and others collaborate intensively to contribute to software projects.
Regression helps predict a continuous quantity. On the other hand, classification predicts discrete class labels, and they can sometimes overlap while working with machine learning algorithms.
Our course instructor
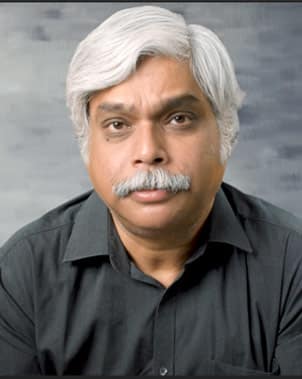
Dr. Abhinanda Sarkar
Academic Director - Data Science & Machine Learning


Ratings & Reviews of this Course
Frequently Asked Questions
Is machine learning a promising career?
Machine learning is a technology that mimics human actions. There is not much workload on human programmers; they are supposed to supervise the machines and give commands to mimic the activities based on previous results. Therefore, machine learning makes an outstanding career.
What level of mathematics is needed to learn machine learning?
Probability, statistics, linear algebra, and calculus make the base foundation for machine learning. A machine learning professional must have good knowledge in working with these sets of mathematical fields.
Will I get a certificate after completing this Introduction to Machine Learning free course?
Yes, you will get a certificate of completion for Introduction to Machine Learning after completing all the modules and cracking the assessment. The assessment tests your knowledge of the subject and badges your skills.
How much does this Introduction to Machine Learning course cost?
It is an entirely free course from Great Learning Academy. Anyone interested in learning the basics of Introduction to Machine Learning can get started with this course.
Is there any limit on how many times I can take this free course?
Once you enroll in the Introduction to Machine Learning course, you have lifetime access to it. So, you can log in anytime and learn it for free online.
Popular Upskilling Programs
Introduction to Machine Learning
Machine learning, abbreviated as ML, is a branch of computer science that deals with the study of computer algorithms capable of automatically improving through experience and the use of data. It is closely related to artificial intelligence. The algorithms in machine learning build a model based on the sample data, known as training data. It does not involve explicitly programming to make predictions or decisions. Machine learning algorithms are applied in a wide variety of applications. It is difficult or unfeasible to build algorithms in traditional ways to accomplish the needs, such as in medicine, email filtering, speech recognition, and computer vision.
Computational statistics is a closely related subset of machine learning focusing on predicting using computer data. However, all machine learning subsets are not computational statistics. Machine learning is served by studying mathematical optimization for methods, theory, and application domains. Data mining explains exploratory data analysis through unsupervised learning. A few machine learning implementations design data and neural networks to mimic the working of a biological brain. It is also called predictive analysis in its application across business problems.
Machine learning programs can perform tasks without the necessity of explicitly programming. It learns from the data fed to the system to carry out the tasks. The system programs algorithms commanding the machine on how it has to execute all the required steps to solve the problem; the computer need not learn anything on its part. It is highly challenging for an individual to manually create the algorithms needed for advanced tasks. It is highly effective and contributes positively to helping machines develop an algorithm based on the requirement.
The machine learning discipline involves different methods to help computers accomplish tasks where convincing algorithms are unavailable. In cases where many potential solutions are available, one approach is used to label a few correct solutions as valid. This data can be used for training purposes for the system to improve the algorithms to determine fitting solutions. For example, when a system is trained to recognize digital characters, the MNIST dataset where the handwritten digits are generally used.
The recent use of machine learning includes two objectives: classifying the data based on the deployed models and making predictions for future experiments or outcomes based on the trained models. A hypothetical algorithm specifically for classifying data uses computer vision models coupled with supervised learning to classify cancerous moles. On the other hand, for the stock exchange, the machine learning algorithm gives data to the trader for future potential predictions.
Optimization
Optimization is closely associated with machine learning; many learning problems are formulated to minimize a few loss functions on training example sets. These loss functions define the discrepancy between the predictions of the trained model and the actual problem instances, such as, in classification, the programmer may want to assign a label to instances, and models are trained to accurately predict the previously given labels of a set of examples.
Generalization
The differentiating point between optimization and machine learning is the goal of generalization; while optimization algorithms potentially minimize the loss on training a data set, machine learning is much focused on reducing the loss on unseen data samples. Characterizing the generalization of different learning algorithms is an active subject of current research, especially in deep learning algorithms.
Statistics
The machine learning field of computer science is closely associated with statistics in terms of methods, but they have unique principal goals; statistics infers population observations from a sample, while machine learning uses generalization prediction patterns. Machine learning ideas have had a long history in statistics, from methodological principles to theoretical tools. There are two statistical modelling paradigms: data model and algorithmic model. Algorithmic models speak closely about machine learning algorithms like Random forest. Some statistics have adopted methods from machine learning techniques, leading to a combined field called statistical learning.
Introduction to Machine Learning course offered to you by Great Learning will help you understand the subject better by walking you through a range of topics like supervised learning, unsupervised learning, classification and regression, various associated subjects like statistics, steps involved in machine learning to a system, case study, applications, and many others. This course is designed to cater to machine learning enthusiasts and help you learn machine learning from scratch. You can enroll in an AI and Machine Learning course to better understand the subjects.