Regression Analysis Using R
Boost your career in Data Analysis by comprehending regression and classification skills. Take up this free course to learn linear regression, multicollinearity, fit-R square and variables concepts for modeling and analyzing data.
Instructor:
Dr. Bappaditya MukhopadyayRatings
Level
Learning hours
Learners
Skills you’ll Learn
About this course
Predictive Modeling and Analytics - Regression is designed to help you better understand the basic concepts and cater to your queries on the subject. The course introduces you to predictive modeling and takes you through simple linear regression, best fit line, multiple linear regression, linear regression assumptions, multicollinearity, fit-R square, and variables concepts. It then discusses predictive modeling classification techniques in the latter part of the course. You will understand each of these concepts with the demonstrated examples and solved problems. You will also be able to judge your gains and test your skills with designed subject-oriented assessments at the end of the course. The course also provides you with materials you can refer to at any time after enrolling.
After completing this free, self-paced, intermediate's guide to Predictive Modeling and Analysis - Regression, you can embark on your Data Science and Business Analytics career with a professional Post Graduate certificate and learn various concepts with millions of aspirants across the globe!
Course Outline
This module shall define predicting, modeling and analyzing and discuss predictive modeling in the first half. The course then speaks about how these predictions can prescribe what tasks to perform to drive the motive of an organization.
This section begins by telling you what a model is. It then continues by helping you understand how simple linear regression is performed with assumption and simplification steps. You will also learn to model an equation based on the given scenario.
This section tells you how one dataset influences the other depending upon the requirements and time. It also talks about how the estimated data can predict the progress and output in the future, through testing and training, by describing a real-life business scenario.
This section describes a supervised learning technique where an outcome results from multiple predictions. You will understand how the result is obtained by deriving the solution to the scenario through multiple linear regression methods.
Here in this module, you will understand some linear regression assumptions, such as the assumption of linearity and the assumption of normality of the error distribution. Lastly, you will understand various dimensions to plot the linear regression models.
Our course instructor
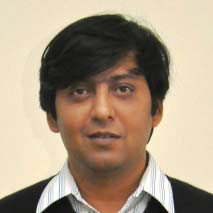
Dr. Bappaditya Mukhopadyay
Professor, Analytics & Finance


With an MA in Economics from Delhi School of Economics and PHD from ISI, Dr. Mukhopadhyay is currently the professor and chairperson of the PGPBA program at Great Lakes Institute of Management. He is also the visiting professor of the University of Ulm, Germany, and distinguished Professorial Associate, Decision Sciences and Modelling Program, Victoria University, Australia. His areas of interest and expertise include applied economic theory, game theory, analytics, statistics, econometrics, derivatives and financial risk management, survey design, execution, and others.
Noteworthy achievements:
- Ranked 4th Amongst the "20 Most Prominent Analytics & Data Science Academicians In India: 2018".
- Prominent Credentials: He has various research papers published in national as well as international journals. He is currently working on a book titled Measuring and Managing Credit Risk. He has been the Managing Editor at Journal of Emerging Market Finance and Journal of Infrastructure and Development, member of Index Committee, member of Research Advisory Committee, Research Advisory Committee, NICR, Expert member in Faculty Selection committees at various Business schools, among others.
- Research Interest: Information economics and contract theory, financial risk management, credit risk and agency theory, microfinance institutions, financial Inclusion, analytics in public policy.
- Teaching Experience: He has more than 20 years of teaching experience in economics, finance.
Ratings & Reviews of this Course
Frequently Asked Questions
What are the prerequisites required to learn this Predictive Modeling and Analytics - Regression course?
This is an intermediate-level course. So before you learn Predictive Modeling and Analytics for Regression modeling, you will need to learn the basics of Data Science to understand the basic terms such as modeling and prediction. Regression is a mathematical concept that solves big data set problems such as pattern matching and prediction.
How long does it take to complete this free Predictive Modeling and Analytics - Regression course?
The Predictive Modeling and Analytics - Regression is 2.5 hours long course but is self-paced. However, once you enroll, you can take your own time to complete the course.
Will I have lifetime access to the free course?
Once you enroll in the course, you will have lifetime access to any of the Great Learning Academy's free courses. You can log in and learn whenever you want to.
What are my following learning options after this Predictive Modeling and Analytics - Regression course?
After completing the Predictive Modeling and Analytics - Regression course, you can learn other concepts in Data Science and apply the subject to solve Business Analytics problems in real life. You can learn various algorithms used to develop and train a model and understand its applications.
Why learn Predictive Modeling and Analytics - Regression?
Predictive Modeling and Analytics - Regression is one of the essential concepts in both Machine Learning and Data Science techniques. It is the procedure to map the patterns to understand the similarities and automate the process. Regression is one of the techniques used to model and analyze the data set.