Principal Component Analysis
Enroll in this free course to understand the important concepts in Machine Learning – Principal Component Analysis, data preparation, and its transformation. Learn hypothesis testing and business analytics through case studies.
Instructor:
Dr. R.L. ShankarRatings
Level
Learning hours
Learners
Skills you’ll Learn
About this course
In this course, you will be introduced to Business Analytics and a hypothesis to understand the concept better. Later, you will learn about important terms employed, such as data collection, specification, and data transformation for analysis purposes. The main focus of the course will be on Principal Component Analysis, popularly transforming large datasets. The hypothesis helps you get familiar with statistical and error concepts in PCA. Lastly, the instructor will brief you with an example of a real-life case study that will help you understand MS Excel's statistical functions.
Do you want to upskill yourself further with Machine Learning? The wait is over with our professional Machine Learning Courses that cover every topic you need to make your career in this domain.
Course Outline
In the introduction part, you will understand Business Analytics in brief. Later, you will understand topics such as data collection and specification of data for the analysis model. Lastly, you will learn to apply algorithms to predict models.
In this module, you will familiarize yourself with two types of hypotheses: Null and alternative. Then you will understand two types of errors that come in the application of these hypotheses. Lastly, you will learn about uniform powerful tests of the hypothesis.
This module discusses how you can apply the hypothesis in various exercises. It also covers various scenarios for applying the Null and Alternative hypotheses.
In this module, you will learn about testing with reference to the previously discussed hypothesis in an example of a Marketing Exam. Using these hypotheses, you will learn to analyze the success and failure of a product in the market.
In the final module, you will understand how you can calculate the probability of Null and Alternative hypotheses for different scenarios using MS Excel. You will also learn some useful statistical functions of Excel.
Our course instructor
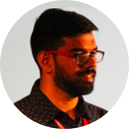
Dr. R.L. Shankar
Professor, Finance & Analytics


Dr. R.L. Shankar is a professor of finance and analytics with over ten years of experience teaching MBA students, Ph.D. scholars and working executives. He has BTech from IIT Madras, MS in computational finance from Carnegie Mellon University, US, Ph.D. in Finance, EDHEC (Singapore), and has trained over 2,000 executives from prestigious firms. With multiple research papers published under his name, he recently received a research grant from NYU Stern School of Business and NSE for original research on Low latency trading and co-movement of asset prices.
Noteworthy achievements:
- Ranked 15th in the "20 Most Prominent Analytics & Data Science Academicians In India: 2018".
- Rated among the" Top 40 under 40" infuential teachers by the New Indian Express.
- Current Academic Position: Professor of Finance and Analytics, Great Lakes Institute of Management.
- Prominent Credentials: He has been a visiting professor at IIM Kozhikode, IIM Trichy, and IIM Ranchi. He is also a TEDx speaker.
- Research Interest: Algorithmic trading, market microstructure, imperfections in derivatives markets and non-parametric risk measurement techniques.
- Teaching Experience: More than 15 years.
- Ph.D. in Finance from EDHEC (Singapore).
Frequently Asked Questions
What are the prerequisites required to learn this PCA course?
There are no special prerequisites required for this course. But it is better to know statistics before you enroll in this course.
How long does it take to complete this free Principal component analysis course?
It will take less than an hour to complete this course as the video content available has the duration of an hour. You can learn the course at your convenience since it is self-paced.
Will I have lifetime access to the free course?
Yes, you can access this course anytime and rewind the lessons at your convenience.
What are my next learning options after this PCA course?
After finishing this course, you can choose the professional Machine Learning course that will help you build your career in the trending field of technology. In addition, you can also go for an Analytics course to learn advanced skills in PCA.
Is it worth learning Principal Component Analysis?
Yes. The principal component analysis is a powerful technique used for the processing of data in Supervised Learning. It is useful for reducing the dimensionality of data, especially for large datasets.
Popular Upskilling Programs
Other Data Science tutorials for you
Principal Component Analysis
Principal Component Analysis is also commonly known as the PCA technique. This technique is vastly known for the reduction in the dimensionality of data sets involving many variables correlated to each other either lightly or heavily while determining the variation present in the data set to the maximum extent. This technique creates a new data set of variables by transforming the existing variables. These newly created variable data set are known as Principal Components. With the creation of these Principal Components, you will see a decrease in the retention of the variations in the original data sets, and it continues to move down in order. Thus, the first Principal Component holds on to the maximum variation that was previously present in the original components.
Principal Components are said to be orthogonal as they are the eigenvectors of the covariance matrix. The data set you intend to use must be scaled to use Principal Component Analysis techniques, and the results are also sensitive to the relative scaling. It is also important to note that this technique comes under the method of summarizing the data. While processing large data, you will encounter redundancies as many of them will measure related properties. The Principal Component Analysis technique will help you summarize small chunks of data from the original data set with fewer factors to consider, reducing redundancies. Through this Principal Component Analysis, the users can have a lower-dimensional picture, a “shadow” or projection of the objects when it is viewed from the most informative viewpoints.
PCA is used for predictive modeling and exploratory data analysis. It is the technique that allows you to deduce strong patterns from the data set by reducing the variances. This technique first looks after the lower-dimensional surface to highlight the high-dimensional data. The Principal Component technique functions by considering the variance of each attribute present, and the high attribute indicates a good split between the classes. This process allows it to reduce dimensionality. Many real-world applications use Principal Component Analysis like image processing, recommendation systems, face recognition, optimizing the power allocation in various communication channels, and many more.
PCA is also known as the feature extraction technique that highlights the essential variables and drops the least critical variables. You will also notice that Principal Component Analysis runs based on some Mathematical concepts like
-
variance and covariance
-
Eigenvalues and Eigen factors
Some of the standard terms utilized in Principal Component Analysis are:
-
Dimensionality : It refers to the number of variables present in the considered data set. You can also take it as the columns present in the data set.
-
Correlation : This signifies the strong relationship between the two variables. You can also say that they are closely dependent on one another.
-
Orthogonal : It signifies that the variables are not correlated to one another, and hence the correlation of these pairs of variables is zero.
-
Eigenvectors : Consider having a square matrix M, and v is the non-zero vector. Then v becomes the Eigenvector if Av is the scalar multiple of v.
-
Covariance Matrix : A matrix is considered a covariance matrix if it has covariance between the pair of variables.
You are already aware that transformed new features are known as the Principal Components. The number of these Principal Components is either less or equal to the original data set features. The properties of these components are as follows:
-
These Principal Components must be the linear combination of the features present in the original data set.
-
The correlation between the pairs of variables is found to be zero, which indicates that these Principal Components are Orthogonal.
-
The values of these Principal Components decrease when moved downwards. It implies that the first Principal Component has the most importance, and once you move down the order, their value decreases, and they are the least important.
Steps involved in Principal Component Analysis are:
-
Arranging the data set
-
Represent the data into a structure
-
Standardize the data
-
Calculate the covariance of Z
-
Calculate the Eigen Values and Eigen Vectors
-
Sort the Eigen Vectors
-
Calculate the new features or the Principal Components
-
Removes less important or unimportant features from the new dataset
In most hypothesis testing, the probability value or the p-value is used to observe the test results or other extreme outcomes, assuming that the null hypothesis (H0) is true. The concept of p-value was introduced by Statistics and is widely used in Data Science and Machine Learning. These p-values play a vital role in hypothesis testing. P-value is also a method of determining their point of rejection to provide the least significant level where the null hypothesis is least considered or rejected. The significance level lies between 0 to 1. The smaller the p-value, the more robust evidence it provides to reject the null hypothesis. The 0.05 p-value is known as the level of significance, and this decision is taken based on the two suggestions:
-
If p-value > 0.05, it shows that the larger the p-value, the stronger the need to accept the null hypothesis.
-
If p-value < 0.05 shows the lesser the p-value, the stronger the need to reject the null hypothesis and declare the result statically significant.
To learn more about PCA analysis, PCA Python implementation, PCA Machine Learning techniques, and to go through Principal Component Analysis examples, enroll in Great Learning’s free Principal Component Analysis course and get a better understanding of its mechanism. Complete the course successfully to attain free Principal Component Analysis certificate that will help you grab better Data Science job opportunities. Enroll Today!