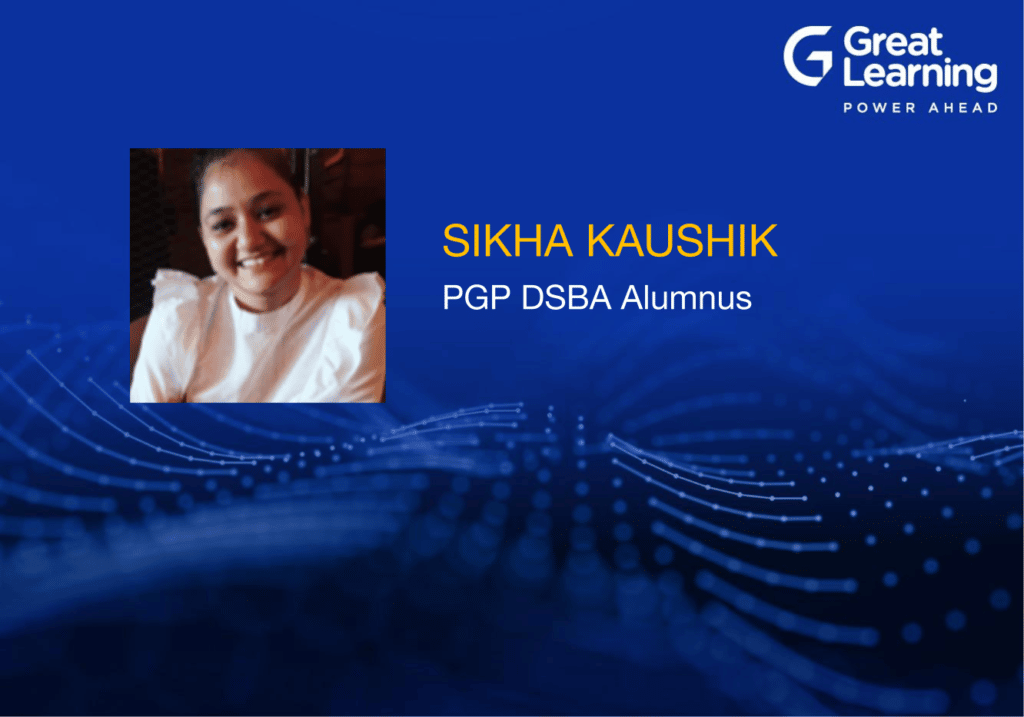
I am from Faridabad, Haryana. I have an academic background in Mathematics and Statistics. My highest qualification includes a Master’s Degree followed by PGP-DSBA Course from Great Learning. My father is an ex-serviceman from the Indian Air Force, so my family used to travel a lot, and hence I believe I love traveling by trekking and hiking. My mother has worked as a teacher for 25 years, and my elder brother is an ERP consultant and Odom Developer. Currently, I am working as a Data Scientist in a Power Utility Company located in Delhi.
Problem Statement
One of the major issues in the utility industry is power theft and hence revenue loss. To tackle this issue, the company has been doing random visits to certain locations and identifying and billing the consumers involved in direct/indirect theft. But this involves a significant investment in the cost of creating an enforcement team, their travel arrangements, etc. As only a small percentage of consumers indulge in theft, identifying them is a difficult task, and hence many times, inspection visits cost much more than what is gained by identifying the cases. To overcome this loss of revenue, the company needs a data-centric approach such that the enforcement team visit locations based on the probability of finding cases of theft in the region and not randomly visit places to avoid loss of money and time.
Solution
One of the solutions proposed by me is picking out consumers through EDA and clustering analysis based on their consumption history and how often they default on bills. Every customer has a different electricity usage, for example, domestic use, Non-Domestic, Industry, Agriculture, Commercial, etc. Also, each customer has a different sanctioned load as per their needs. After anomaly detection in EDA, it is observed that a customer has often taken a domestic connection type but is using the connection for a small business or running industry to avoid charging electricity for industry. This is a particular kind of theft as their maximum demand indicator (MDI) value is higher than their Sanctioned load. Another observation made using K-means clustering is that consumers’ MDI is significantly low compared to their Sanctioned load. This means the consumer is not utilizing the complete load sanctioned and has very little consumption compared to other consumers of similar connections in its cluster. These kinds of cases are potential theft cases and must be inspected.
Similarly, we have 3 clusters based on their type of connection and consumption, etc. We pick up outliers from each of these clusters and do a sample check from them to understand and verify their pattern of behavior. I presented my findings and methodology to my manager and handed over such cases’ information to the enforcement team to investigate.
Impact
The proposed system was accepted by the manager and the enforcement team. The proportion of finding theft cases out of all the cases handed to the team improved by 30%. This is making a good impact at the organizational level to detect, investigate, and book theft cases. This not only increased my morale as a contributor, but of the whole team, as their efforts in the investigation were not getting wasted.