Free Data Science Foundations Course
Data Science Foundations
Enrich your skills in Data Science by strengthening your foundational knowledge. Enroll in this free course and thoroughly learn the life cycle, tasks, programming languages, and analytics landscape concepts.
Instructor:
Dr. Bappaditya MukhopadyayRatings
Level
Learning hours
Learners
Skills you’ll Learn
About this course
The Data Science Foundations course roffers your knowledge on the introduction to the subject and gives you insights into the different phases of its life cycle. The course covers topics about various tasks carried out in Data Science and different programming languages that are compatible to work with to accommodate the tasks efficiently, and Machine Learning, contributing to the dynamic behavior of machines and making significant associations with DS. The analytics landscape is another significant component within an organization, which you will learn in the latter part of the course, to understand workflow and asset distribution thoroughly. You will have to take an assessment to test your gain on the subject. The course also provides you with study materials for your reference at any given point after enrolling in it.
After this free, self-paced, beginner's guide to Data Science Foundations, you can embark on your Data Science career with the professional Post Graduate certificate and learn various concepts in depth with millions of aspirants across the globe!
Course Outline
This section gives you various examples to help you understand Data Science. It explains how you decide on a place for the vacation, how the weather is predicted, and sales during a particular time in a year using data science.
Data Science life cycle revolves around data acquisition, preprocessing, ML algorithms, pattern evaluation, knowledge representation, and analytical strategies to predict and proffer insights, which we shall learn in this module, into the procedures to yield the best results.
Data mining tasks include classification, prediction, association, clustering, and summarization. This module explains anomaly detection, continues with matching data points and explains the concept with a real-life example.
Machine learning is a learning method to process raw data based on the previously trained model for similar input data. This section explains how machines understand the patterns and the features through which it tags every data.
Most commonly used programming languages used in Data Science are R (for statistical computation) and Python (including MATLAB library), which we will know why in this section. We will also look into the famous libraries in each of these languages for Data Science.
Our course instructor
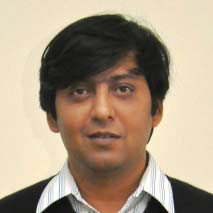
Dr. Bappaditya Mukhopadyay
Professor, Analytics & Finance


With an MA in Economics from Delhi School of Economics and PHD from ISI, Dr. Mukhopadhyay is currently the professor and chairperson of the PGPBA program at Great Lakes Institute of Management. He is also the visiting professor of the University of Ulm, Germany, and distinguished Professorial Associate, Decision Sciences and Modelling Program, Victoria University, Australia. His areas of interest and expertise include applied economic theory, game theory, analytics, statistics, econometrics, derivatives and financial risk management, survey design, execution, and others.
Noteworthy achievements:
- Ranked 4th Amongst the "20 Most Prominent Analytics & Data Science Academicians In India: 2018".
- Prominent Credentials: He has various research papers published in national as well as international journals. He is currently working on a book titled Measuring and Managing Credit Risk. He has been the Managing Editor at Journal of Emerging Market Finance and Journal of Infrastructure and Development, member of Index Committee, member of Research Advisory Committee, Research Advisory Committee, NICR, Expert member in Faculty Selection committees at various Business schools, among others.
- Research Interest: Information economics and contract theory, financial risk management, credit risk and agency theory, microfinance institutions, financial Inclusion, analytics in public policy.
- Teaching Experience: He has more than 20 years of teaching experience in economics, finance.
What our learners enjoyed the most
Skill & tools
60% of learners found all the desired skills & tools
Ratings & Reviews of this Course
Frequently Asked Questions
Is the Data Science Foundations course a certification course?
What are the prerequisites required to learn this Data Science Foundations course?
You do not need any prior knowledge except knowing what computer science is to learn the Data Science Foundations course. But suppose you want to do a little homework to understand the concepts of Data Science faster. In that case, we recommend you learn algorithms used to work with Data Science since you can implement them in any programming language.
How long does it take to complete this free Data Science Foundations course?
Data Science Foundations is 2 hours long course but is self-paced. Once you enroll, you can take your own time to complete the course.
Will I have lifetime access to the free course?
Yes, once you enroll in the course, you will have lifetime access to any of the Great Learning Academy’s free courses. You can login and learn whenever you want to.
What are my next learning options after this Data Science Foundations course?
The free Data Science Foundations course is a head start to learning Data Science concepts, its working, various processes and approaches, its applications, and Machine Learning. So you can continue with your learning journey by enrolling in a professional Data Science and Machine Learning course.
Popular Upskilling Programs
Other Data Science tutorials for you
Data Science Foundations
In the digital age, data has become the lifeblood of organizations, driving decision-making, optimizing processes, and delivering valuable insights. Data science, a multidisciplinary field at the intersection of statistics, mathematics, computer science, and domain knowledge, plays a pivotal role in extracting meaningful information from vast datasets. At its core, data science relies on foundational concepts that provide the necessary framework for understanding, analyzing, and interpreting data. Let's explore some of these key pillars that form the bedrock of data science.
Data Collection and Preprocessing:
Data science begins with data acquisition, a process that involves collecting and assembling data from various sources, such as databases, APIs, websites, or even physical sensors. This data is often heterogeneous and noisy, necessitating preprocessing steps to clean, transform, and organize it into a structured format. Data cleansing, dealing with missing values, and handling outliers are vital steps to ensure the quality of the data before proceeding with analysis.
Statistics and Probability:
Statistics serves as the backbone of data science, enabling professionals to summarize and interpret data, identify patterns, and draw inferences. Probability theory provides the basis for uncertainty modeling, essential in situations where data is noisy or incomplete. Techniques such as hypothesis testing, regression analysis, and Bayesian inference are commonly employed to make data-driven decisions and predictions.
Machine Learning:
Machine Learning (ML) algorithms empower data scientists to build predictive models and discover patterns in data. Supervised learning deals with labeled data, where the algorithm learns from past examples to make predictions on new data. Unsupervised learning, on the other hand, involves finding patterns in unlabeled data, enabling clustering and dimensionality reduction. Reinforcement learning is another branch, where agents learn from their interactions with an environment to optimize their actions and achieve specific goals.
Data Visualization:
Visual representation of data is a powerful tool to communicate complex information effectively. Data visualization allows data scientists to explore patterns, trends, and relationships, making it easier for stakeholders to grasp insights and make informed decisions. Graphs, charts, heatmaps, and interactive dashboards are just a few of the visualization techniques used in data science.
Programming and Tools:
Proficiency in programming languages is crucial for data scientists to manipulate data and implement algorithms. Python and R are two of the most popular programming languages in the field, offering extensive libraries for data manipulation, analysis, and machine learning. Additionally, data scientists leverage various tools and frameworks, such as TensorFlow, PyTorch, and scikit-learn, to simplify the development and deployment of ML models.
Big Data and Distributed Computing:
The increasing volume and complexity of data necessitate the use of big data technologies. Distributed computing frameworks like Apache Hadoop and Apache Spark enable the processing of massive datasets across multiple nodes, enhancing scalability and performance. Data scientists need to be familiar with these tools to handle big data challenges efficiently.
Data Ethics and Privacy:
As data science becomes more pervasive, ethical considerations and data privacy gain prominence. Data scientists must be conscious of the potential biases present in the data and the ethical implications of their analyses. Ensuring data privacy and complying with data protection regulations are essential to maintaining public trust and safeguarding individuals' sensitive information.
In conclusion, data science is an ever-evolving field that relies on strong foundational principles. The ability to collect and preprocess data, understand statistics and probability, apply machine learning algorithms, visualize data effectively, program efficiently, handle big data, and prioritize data ethics are essential skills for any data scientist. By mastering these foundations, data scientists can unlock the true potential of data and drive valuable insights that foster innovation and informed decision-making across various industries.